How Qualitative Data Analysis is Like Solving a Puzzle
Written by Dr. Susanne Friese
"Coding means that we attach labels to segments of data that depict what each segment is about. Through coding, we raise analytic questions about our data […]. Coding distills data, sorts them, and gives us an analytic handle for comparisons with other data segments." (Charmaz, 2014: 4)
"Coding is the strategy that moves data from diffuse and messy text to organized ideas about what is going on.’" (Richards and Morse, 2013:167)
Coding is a core function in computer-aided qualitative data analysis software (CAQDAS) software that lets you ‘tell’ the software where the interesting things are in your data. Coding, in a technical sense, simply means assigning a label to a data segment. A better-known term these days is tagging. The goal of tagging is to find the things you tagged using the tag name. The software uses the words ‘code’ and ‘coding’, as most other CAQDAS do. I guess that this is because of the popularity of grounded theory at the time when the first programs were developed in the late 1980s and early 1990s. Coding in CAQDAS, however, is very different from grounded theory coding in the methodological sense (see Friese, 2016 and 2019). If you are more comfortable with the idea of tagging, in what follows, simply replace the terms ‘code’ and ‘coding’ in your mind with ‘tag’ and ‘tagging’.
A code in the software can be a simple description, a concept, a category, a subcategory, or a wildcard that modifies a link in a network. The software itself does not dictate how to use a code. It only provides this entity as an item in the toolbox. To better understand what coding data means, I would like to play a virtual puzzle with you. You probably have played a puzzle before at some point in your life. You can apply the skills you learned when playing puzzles to coding qualitative data.
Coding qualitative data and playing a puzzle – what have the two in common?
Imagine you are sitting at a table. On the table, there are 1,000 parts of a jigsaw puzzle with the picture side up (Figure 1). Now it's your turn. Your task is to put the puzzle together. How do you go about it?
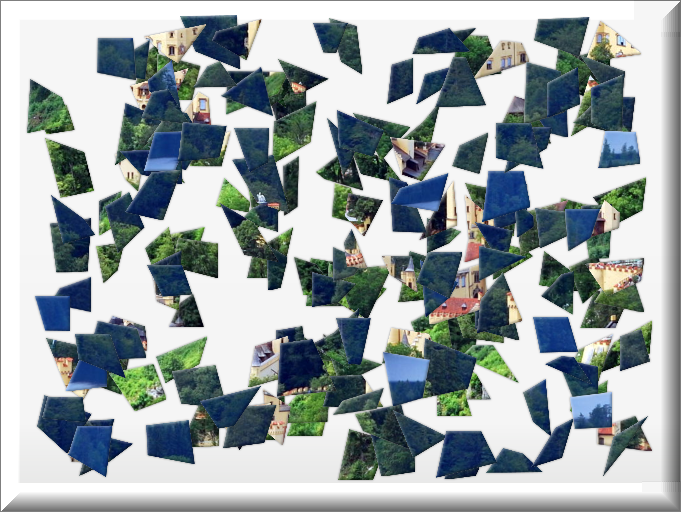
Most people would answer that either they begin with the corners and the edges or they sort by colors or shapes. Let’s begin with the corners and edges. Why do you think that most people begin like that? These pieces are easy to recognize since they have at least one straight edge. When it comes to analyzing a project in ATLAS.ti, I likewise recommend you begin with what is easiest. See also "Preparing Data for Analysis in Popular QDA Software".
By starting to work on a project in this way, you have literally framed it (Figure 2).
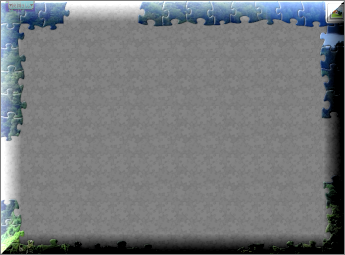
As soon as the frame is laid, the next task is to examine the other pieces in the puzzle. You could try to find those parts that belong together. But that's probably tedious. A better strategy is to sort the parts by color and similarity in terms of what is visible on them (Figure 3). The sample puzzle here depicts a castle with a forest around it and a lake in the upper-right-hand corner (I know this as I have seen the lid of the box).
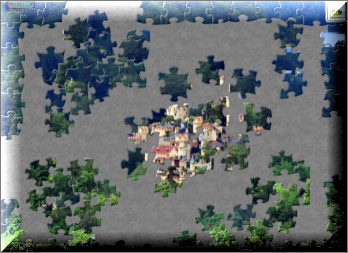
The next step is to take a closer look at one of the piles. Let's look at the pieces of the puzzle that look like they belong to the castle. Approaching this strategically, one looks for puzzle pieces that belong to a part of the castle, like the roof, the towers, the windows or the battlements. In other words, one segments the castle into sub-units (Figure 4). This process is repeated for all pre-sorted stacks until everything can be put together to complete the puzzle.
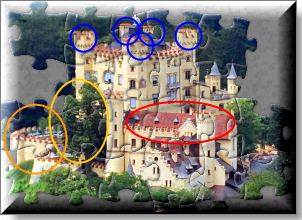
Pre-sorting the parts of the puzzle into piles of similar elements is like coding the data by major themes. Segmenting the piles into smaller sub-units is like building subcategories. Even if you are not a seasoned puzzle solver, just growing up, you acquired skills in everyday life that you can apply to construct categories and subcategories. You do it every day, and you learned the technique a long time ago when discovering the world as a child. You may have first realized that a certain animal is a dog and then used the word ‘dog’ for the various kinds of dogs. Later you learn they are beagles, boxers, golden retrievers, poodles, mongrels, etc. Developing subcategories for your data is not much different.
If you have collected many quotations under a common label, the next step is to look through them, as I did with the castle pieces. After reading or looking at a few quotations, you will quickly notice where the commonalities are. Having coded the data, the software makes it easy to retrieve all segments that belong to one topic and take a second, closer look. The aim is to develop subcategories to bring some order to the pile and differentiate the various aspects of the topic area you are looking at.
Differences between playing a puzzle and coding in qualitative data analysis
Having pointed out the similarities between playing a puzzle and coding qualitative data, I should say there are also some differences. Qualitative data are not yet broken down into parts. This happens in ATLAS.ti when you create quotations. This can be done while you code or as a distinct analytic step before coding. This is for instance, very useful when working with video data.
Often when playing a puzzle, the individual parts are initially sorted into larger clusters. When coding data, the main topics may not be so obvious, and it is a process to develop them. Sometimes one encodes at the level of subcategories or, even lower, at the descriptive level. And only over time does it become clear which codes belong to a higher-order category.
If you generate lots of codes, i.e. if you quickly have 500 or more codes, be aware that you are basically only naming each piece of the puzzle. You are not sorting and organizing your data yet. If you notice that you are doing this, you should pause and stop coding. Analyze which of the codes can be aggregated so that more data segments can be collected in them. Technically, this means you will be merging codes. The goal is to sort and organize the data rather than just naming each puzzle element.
When you play a normal puzzle, the lid shows you what the finished puzzle should look like in the end. By comparison, in a qualitative research study, we usually do not have a template that shows what the result should be.
The researcher probably has some ideas based on existing literature. However, the answers to the research questions will only emerge through the analysis process. There is a certain kind of puzzle that is similar in strategy to the qualitative analysis approach: the so-called WASGIJ puzzle (this is ‘jigsaw’ backward). The finished puzzle does not match the picture on the box. Instead, the player must assume the role of one of the persons on the cover and put himself in the place of that person. The final image corresponds to what this person sees from his or her perspective. The solution thus results from the process of putting together the parts.
There are also jigsaw puzzles without a template for advanced players with experience. Those puzzles are comparable to a project where it is difficult to find existing literature or earlier research on the subject matter. Thus, you may have a challenging time developing detailed research questions based on previous knowledge, and the only option you have is to go into the field and start collecting data. Grounded theory studies often are like this. Like a puzzle without a template, such a project is proportionately more difficult than one that is guided by research questions. First ideas for coding can be derived from research questions, theories, the literature, or the interview guidelines. Ideas for coding in the grounded theory sense can also emerge from the data, but this is not so easy for a beginning researcher. As Kelle and Kluge note:
Novices in the field of social research have a particularly tough time following recommendations like: “let theoretical concepts emerge from your data material”. For them, such attempts might result in drowning in data material for months (2010: 19).
Therefore, I recommend that you do not start with the most challenging kind of puzzle (i.e. methodical approach) if qualitative data analysis is new to you. Instead, use thematic analysis if you are just starting out.
First steps in developing a code system
Unless you want to code deductively using an existing framework, keep an open mind when you begin to code your data, notice as many things as you can, and collect them via coding. If you feel that it is important to read all the data first and to write down notes on a piece of paper before you create codes in the software, then this is a suitable way to proceed. If, however, after reading some of your data, you already have some ideas for codes, go straight ahead and start coding. Do whatever feels most natural to you.
At first, you will generate lots of new codes; in time, you will reuse more and more of the ones you already have, and you won’t need to create new ones. You have reached the first saturation point. In technical terms, this means you will drag and drop existing codes from the Code Manager or navigation panel onto the data segments. At this stage, you have roughly described the various elements in the data. As soon as you reach this point – that is when you no longer add new codes (or only a few) and mostly use drag-and-drop coding – it is time to review your coding system. If you do it at a much later stage, it will need more work because then you will have to go through all the documents again to apply newly developed subcategories and recheck all other codings. I recommend that for this first phase, you work on those documents in your data that are most different. This way, it is more likely that you come across the bandwidth of topics.
Let’s assume you have taken your first coding round up to this point. Those coders who naturally develop a mix of descriptive and abstract codes will have around 100 codes, depending on the project. Smaller student projects may hold around 50–70 codes. The cleaning up and restructuring of a first code list is done within the software.
If you have noticed many things – let’s say you already have 300 or more codes after coding a few interviews – your codes are probably very descriptive. Coders of this type are referred to in the literature as splinters (Guest et al., 2012; Bernard and Ryan, 2010). If you are a splinter, you need to stop coding new data at this point, review your coding and begin to merge your codes. As a splinter, you may struggle to let go of your codes through merging for fear of losing something. I can assure you that this is not going to happen. After merging and reorganizing your codes, you will have a single code that might hold ten quotations in their original form. This is far better than ten codes that only summarize one data segment each (= one piece of the puzzle). The need to push codes from a descriptive to a conceptual has also been described by Corbin and Strauss:
One of the mistakes beginning analysts make is to fail to differentiate between levels of concepts. They don’t start early in the analytic process differentiating lower-level explanatory concepts from the larger ideas or higher-level concepts that seem to unite them. … If an analyst does not begin to differentiate at this early stage of analysis, he or she is likely to end up with pages and pages of concepts and no idea how they fit together. (2008: 165)
This also applies to computer-aided analysis, although it is no problem for the computer to manage 1,000 or more codes. Instead of being conducive to your analysis, a high number of codes prevents further analysis. Creating too many codes is one of the dangers you will encounter on your journey to learning qualitative data analysis.
Building categories and subcategories
The first categories that you develop are likely to be provisional, as they are based on very little coding. With more coding, they are likely to change and develop further. I like Saldaña’s idea of first-cycle and second-cycle coding (Saldaña, 2013: 8). This idea fits the nature of the N-C-T model, where you have seen that qualitative analysis is cyclical rather than linear. First-cycle coding, according to Saldaña (2009: 45), refers to those processes that happen during the initial coding. These are the ideas you notice and collect when you begin the coding process. Second-cycle coding is the next step. From experience, I would like to add that there is at least a third or fourth coding cycle. When coding data, the aim of this process is to develop a structured code list based on a subsample of your data. Once you have developed a first structure, you can apply the codes to the remaining data.
Deepen your understanding of computer-aided qualitative data analysis. Join our upcoming workshops or inquire about tailor-made training for your team. Discover more at Qualitative Research Training.
If you are looking for support: Join the Qualitative Research Community.
Cite as follows:
Friese, S. (2023). "Learning Qualitative Data Analysis: How Qualitative Data Analysis is Like Solving a Puzzle." Dr. Susanne Friese's Blog. Available at: https://www.drsfriese.com/post/cracking-the-code-how-qualitative-data-analysis-is-like-solving-a-puzzle
References
Bazeley, Pat (2013). Qualitative Data Analysis: Pratical Strategies. London: Sage.
Bazeley, Pat and Richards, Lyn (2000). The NVivo Qualitative Project Book. London: Sage.
Bernard, Russel H. and Ryan, Gery W. (2010). Analysing Qualitative Data: Systematic Approaches. London: Sage.
Charmaz, Kathy (2006/2014). Constructing Grounded Theory: A Practical Guide Through Qualitative Analysis. London: Sage.
Corbin, Juliet and Strauss, Anselm (2008/2015). Basics of Qualitative Research: Techniques and Procedures for Developing Grounded Theory (3rd and 4th ed.). Thousand Oaks, CA: Sage.
Fielding, Nigel G. and Raymond, M. Lee (1998). Computer Analysis and Qualitative Research. London: Sage.
Friese, Susanne (2019). Grounded Theory Analysis and CAQDAS: A happy pairing or remodeling GT to QDA? In Tony Bryant and Kathy Charmaz (eds.), chapter 11. The SAGE Handbook of Grounded Theory. London: Sage.
Friese, Susanne (2016). CAQDAS and Grounded Theory Analysis. MMG Working Paper 16-07, October 2016.
Guest, Greg, Kathleen M. MacQueen, and Emily E. Namey (2012). Applied Thematic Analysis. Los Angeles: Sage.
Kelle, Udo und Kluge, Susann (2010). Vom Einzelfall zum Typus: Fallvergleich und Fallkontrastierung in der qualitativen Sozialforschung. Wiesbaden, VS Verlag.
Kuckartz, Udo (1995). Case-oriented quantification, in U. Kelle (ed.), Computer-Aided Qualitative Data Analysis: Theory, Methods and Practice. London: Sage. pp. 158–66.
Richards, Lyn (2009, 2ed). Handling qualitative data: a practical guide. London: Sage.
Richards, Lyn and Janice M. Morse (2013, 3ed). Readme first: for a user’s guide to Qualitative Methods. Los Angeles: Sage.
Saldaña, Jonny (2009/2013/2015). The Coding Manual for Qualitative Researchers. London: Sage.
Silver, Christine and Lewins, Ann (2014, 2ed). Using Software in Qualitative Research: A Step-by-step Guide. London: Sage.
Comments